Blog
How to localize at scale using AI product discovery
This is a new way to do your ecommerce localization at scale. With Klevu, retailers are able to serve hyper-relevant content to their customers in their local language.
Watch the video of this article on YouTube >
The complexity of ecommerce globalization
Businesses expanding into new international markets have to jump through many hoops – relevancy of content languages, currencies, supply chain management, tax, centralized teams running either regional websites or disparate teams, payments, shipping and logistics, environmental compliance and packaging laws, geopolitical status, in-market customer support, and this is only a snapshot of the considerations.
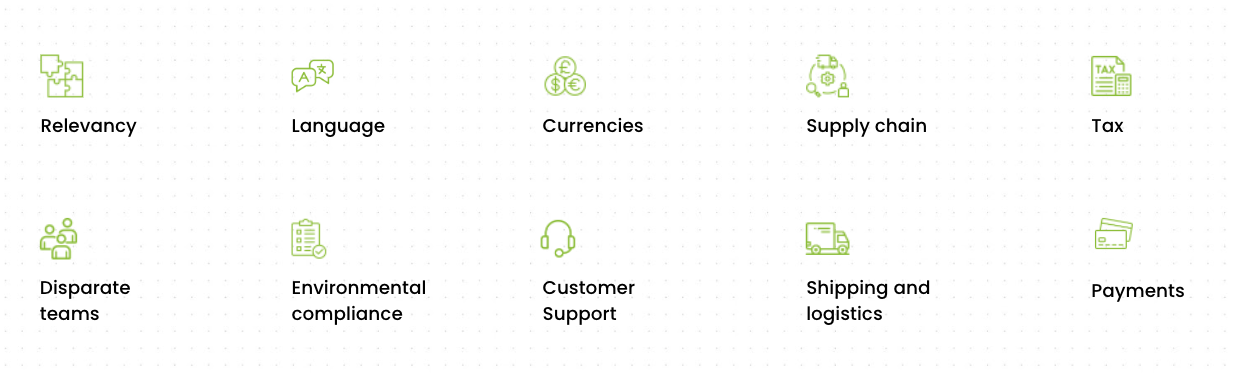
And with all of this, there is very little margin for error. And with all the challenges ecommerce teams face, customers are still expecting excellence.
75% of people want to purchase products in their native language, and this is actually more important to them than the price of the products themselves. 92% of online shoppers prefer to make purchases online in their local currency.
Shoppers in different countries behave differently. But all are looking for hyper-relevant content personalized to them and will bounce from a website that isn’t relevant.
Whether that’s an irrelevant language or an irrelevant brand being merchandised to them.
But, there is hope. There are some retailers doing product discovery well, and they aren’t working lots of overtime or hiring hundreds of hands to do it.
Brands globalizing at scale, with ease
Flying Tiger’s French site has different product recommendations than what they have on their Finnish sites. Using a tool like Klevu, the recommendations banners in different regions auto merchandise from insights from shopper intent, plus whatever business rules they set.
Flying Tiger is able to process 21 different languages, including more advanced queries. In the examples here, you can see in English, that we’re searching for bags on the five. And then we’re searching for a similar query in the French language and we’re still able to serve the most relevant products.
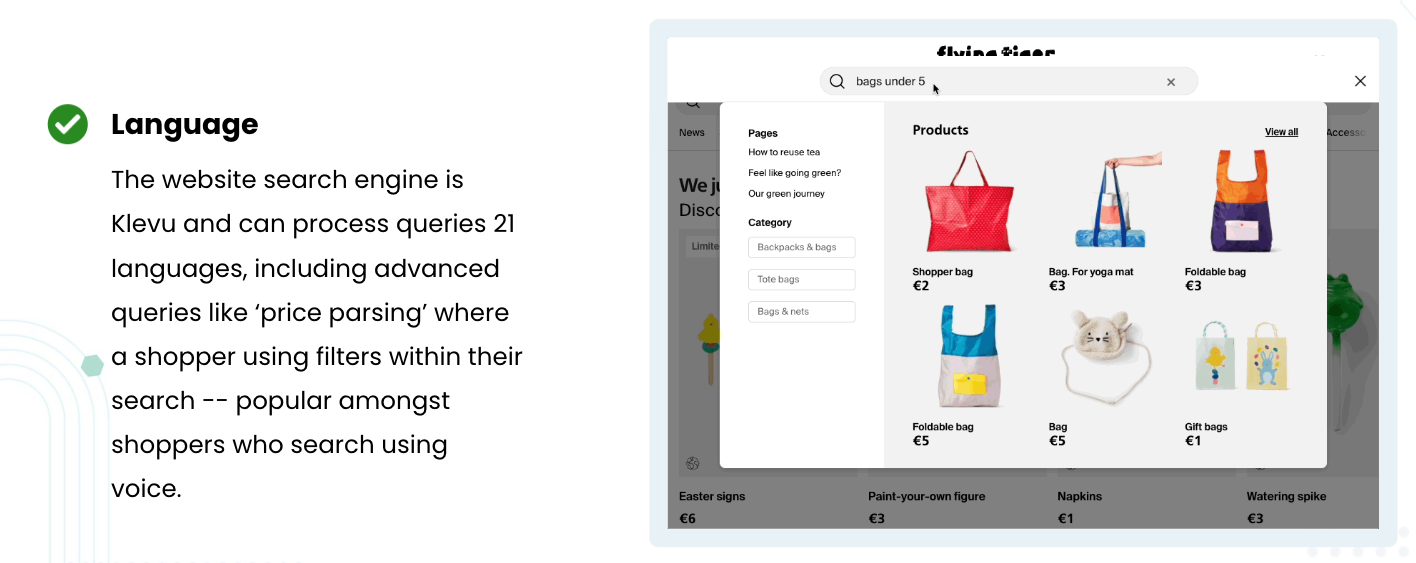
And the way Klevu’s Site Search works when breaking down these types of queries is by understanding the word-level category. So we understand that “bag” is a noun “under” a preposition. And we understand that the price is actually an attribute and we’re able to filter down in such ways.
Level Shoes, a Klevu client based in Dubai localizes products by region – merchandising the categories differently depending on the territory because of local market preferences or brands.
As you can see here on the gifting page, UAE differs from Oman. With the insights that Klevu provides, they are able to automatically show which brands are resonating best with target audiences.
How are these businesses achieving ecommerce website localization processes at scale?
1. Machine learning and user behavior analytics within a local store context
Klevu doesn’t learn across all the sites and generalize the machine learning, it learns on an individual store level, which is essential to understand customer behavior in different territories.
Shoppers in the US behave differently to shoppers in the UK or APAC, and Klevu AI is equipped to compartmentalize local online shopping intent, and reflect that in the results and content that it displays on category listing pages, search engine results, and product recommendation banners.
2. A/B Testing of merchandising strategies in different markets
Because of these local differences in preferences, it’s not wise to roll out one strategy for a global online business. And so, within Klevu, ecommerce teams can complete split A/B tests and understand whether, for example, AI might be working better against visual merchandising or rule-based campaigns, or manual merchandising. This helps fine-tune merchandising strategy, in line with the business logic that they want to apply in different territories.
3. Ability to copy merchandising rules
So whilst we do have the capability to have different online stores behaving differently with our AI merchandising, we are also able to copy merchandising rules across all stores. So let’s say one of our merchandising teams has a global campaign that they want to roll out to all their sites, there’s a really easy way to do that within Klevu’s Merchant Center.
4. Merchandising rules by country
Klevu is able to apply different business logic to specific store contexts. And finally, multilingual NLP really understands the crux and the intent behind the query across different languages.
Advanced Multi-lingual Product Discovery
Some of the key Klevu AI features that dramatically improve multilingual search include:
- Data enrichment – Klevu enriches and normalizes product catalog data automatically
- Semantic Query Processing – Klevu processes and understands what the shopper means.
- Decompounding – Klevu can break complex words into their simplest forms to process them clearly.
- Price Parsing – Klevu comprehends price relativity from a search query.
- Dimensional Transformation – Klevu automatically normalizes different weights and measures.
- Contextual self-learning – Klevu learns within the context of a local store.
Learn more about how Klevu AI works >
So how many languages does Klevu support? The short answer is almost all. Features such as self-learning, error tolerance, etc, are language-independent and available for all of the languages, whereas some of the NLP features such as handling inflections or de-compounding are available for a few languages as of today, however, our team of AI scientists is in the process of making them all language-independent through deep learning AI methods.
Benuta, a global retailer selling household items handles complex queries very well. For example, a query was fired such as the following “brownish circular rungs 115 centimeters in jute material”, here as a result of inflection normalization.
The word “brownish” is automatically associated with the word “Brown.”
The word circular does not have an exact match in the catalog, but because we have automatically added synonyms, the word round was added as a synonym of the word circular making it possible to match to find the products where the term around is specified.
The real word is “rug” however, as we can see in the query, the customer has made a mistake and the customer has entered “runs”. Now, because of the fuzzy matching logic, the word rug is matched with the word “runs” 100 and centimeter 115 centimeter which is recognized as a measurement and also matched by our algorithm.
Finally, the system understands that jute is the material here and it will try to find the products where the material “jute” is also specified.
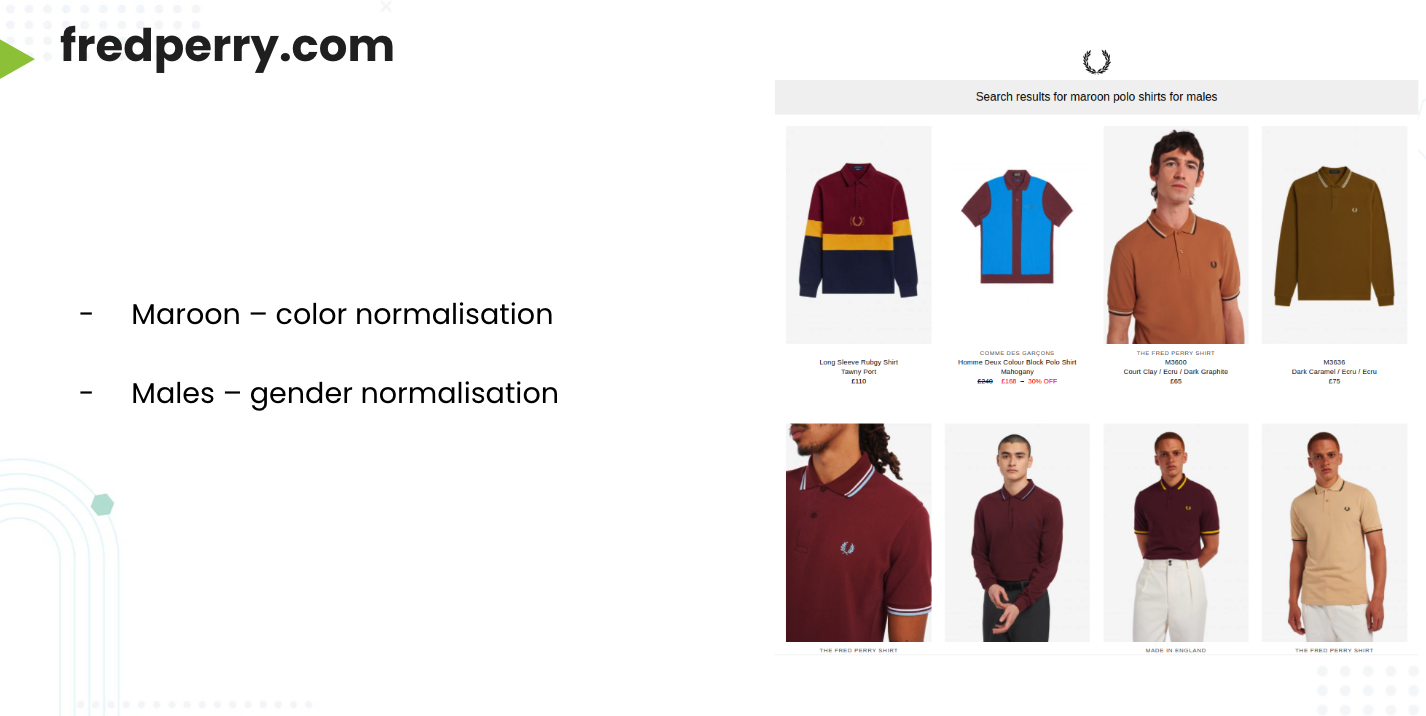
On Fred Perry, we have enabled our special component, which we call color normalization to bring back similar colors when a customer is searching for words such as “maroon” which may not be present in the catalog.
Another component which is gender normalization has also been used. So, when the customer is searching for “males” as a term, it automatically matches with the term “men’s” which is the gender attribute value.
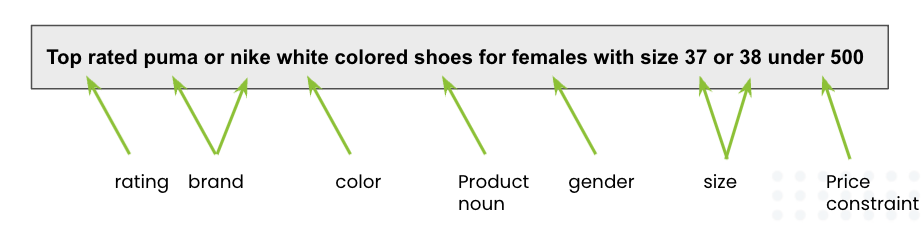
Here is another very complex example, a query is ‘top-rated Puma or Nike white colored shoes for females with size 37 or 38 under 500′ can be seen this is a complex query where multiple features constraints have been specified Klevu is intelligent enough to automatically recognize them and actually apply them when such a query is fired.
These types of queries are very common when somebody is using a voice search or they may want to develop a conversational assistant.
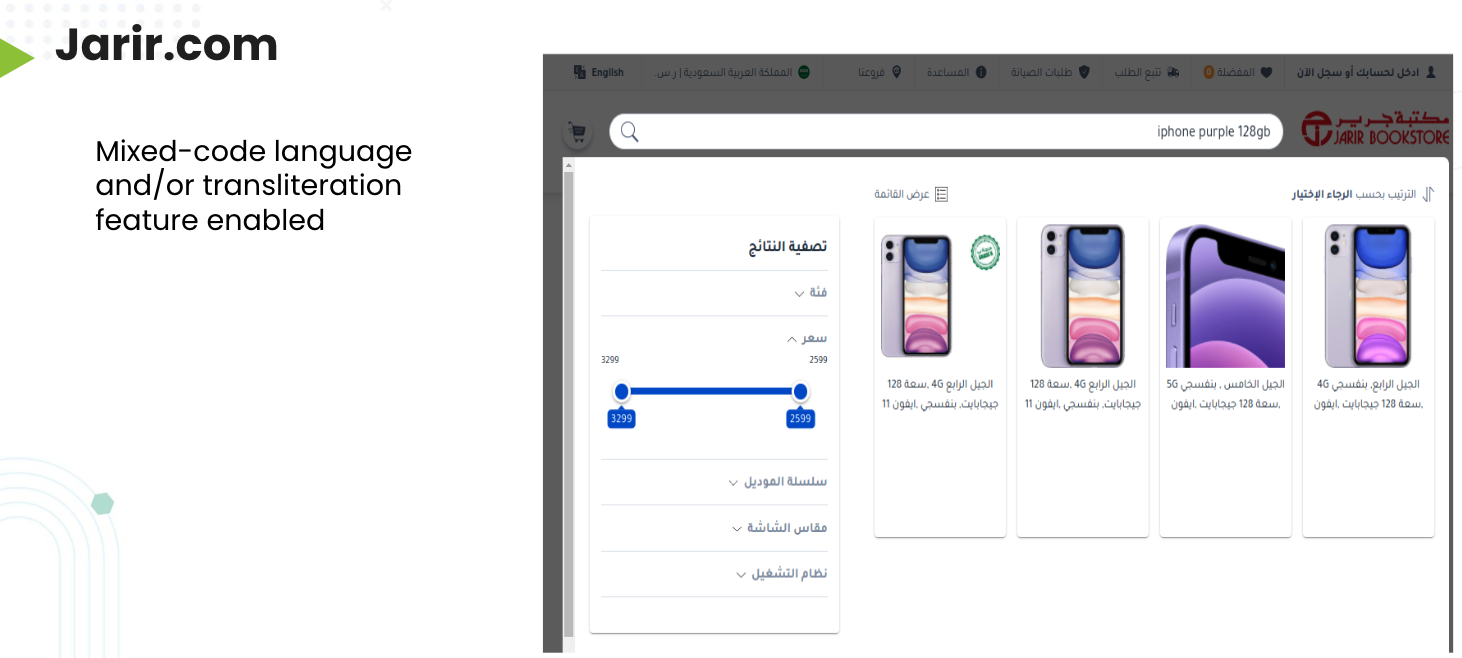
Jarir, is based out of Dubai, and they have many non-Arabic speaking customers, so they had a requirement to allow transliteration in search. In other words, when a shopper types in English, it should match their respective Arabic words. The Klevu team worked together with them to install the library of transliterations to make this possible.
Japan Center has a mixed language catalog, so by typing in ‘陶製 ネコ’ which means ‘Ceramic cat’, it’s then possible to narrow down the search using English.
Key takeaways
Localization with the right content, product, and language doesn’t have to be difficult.
Investing in agile product discovery technology will save you time and hassle when it comes to globalizing at scale and localizing content, product, and merchandising.
Language and cultural preferences are so nuanced, that let the AI learn from your local shoppers and present the right content for them.
Search is complicated! Building your own, having to constantly add synonyms to tweak algorithms becomes unmanageable (and possibly irrelevant) very quickly when scaling globally.
Merchandising on a regional basis will help your conversion, and using A/B testing by region will help your team know for sure what’s working and what isn’t.
Klevu’s unique strengths in linguistics and advanced AI makes it easy to meet the unique needs of your shoppers.
Ready to learn more about how Klevu can support your multi-lingual and globalization strategies? Schedule a demo >