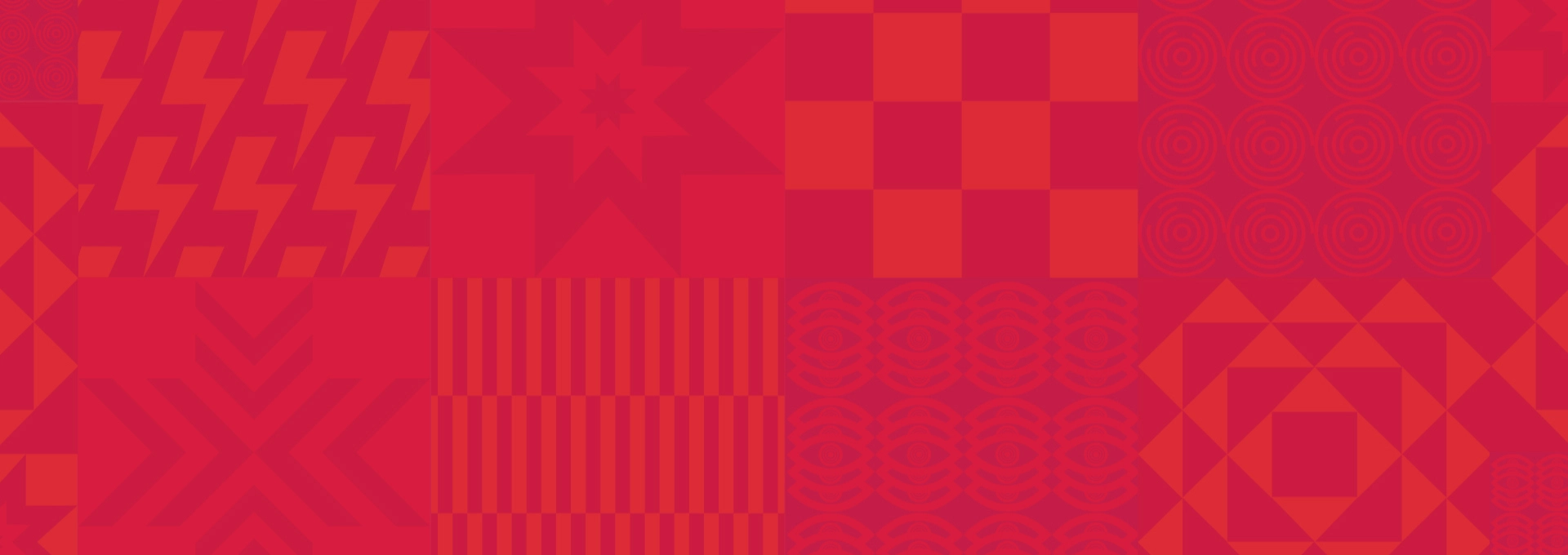
Podcast
Episode 4: Exploring the Evolving Landscape of Artificial Intelligence: From ChatGPT to Conversational AI and Beyond
Join us in this insightful episode as we delve into the current state of Artificial Intelligence (AI) and its exciting future.
Podcast description:
At Klevu’s Discovered London event, Niraj Aswani, Co-Founder and CTO of Klevu, shares his expertise on the transformative power of AI models like ChatGPT and the potential they hold in natural language processing. We explore the different types of search, from keyword-based to neural and semantic search, and how they revolutionize search engine results.
While Chat GPT is one of the most well-known AI models, there are hundreds of other models available, each with its own unique strengths and applications. The Hugging Face company has over 100,000 models in their repository built by the open-source community. With so many models available, the future of AI is likely to involve combining these models to create even more powerful systems.
“As more businesses adopt these technologies, we may see a future where there are only two types of companies: those that have adopted AI and those that do not exist” – Niraj said. While there are limitations and concerns to address, the future of AI is exciting and promising. By learning how to ask questions to these machines, we can make the most of their incredible capabilities.
This podcast episode is perfect for those who want to delve deeper into AI or are beginners on their journey of learning about it.
Guest BIO:
Niraj Aswani, CTO and Co-Founder, Klevu
Niraj has many years of experience in the field of semantic search and natural language processing. He leads the Klevu AI Lab and is one of the brains behind the core Klevu engine that powers millions of searches and recommendations every day.
References:
- Klevu MOI: https://www.klevu.com/moi/
- Hugging Face: https://huggingface.co/
- TensorFlow: https://www.tensorflow.org/
- PyTorch: https://pytorch.org/
- DELL-E 2: https://openai.com/dall-e-2
Transcript:
[00:00:00] Niraj Aswani: Good morning. Thank you.
[00:00:01] I’m one of the co-founders and CTO at Klevu. My background is actually in the field of AI. I’ve been in this since 2002, and since we started the company, AI has been, it has been playing a major role in the technology.
[00:00:16] Today I will be mainly talking about where we are with AI and where we are heading to. We all have been hearing a lot about chatGPT and the models behind it. I think it’s important to understand how have we reached this moment. And what it means to run these models in a large cloud infrastructure.
[00:00:38] So before we get into those points, I thought there are some vocabulary which is going around. People have been talking a lot about chatbot vector embedding. I thought it’ll be good if we can just go through it and, and understand them in a very simple language, what they mean, [00:01:00] because we are dealing in search.
[00:01:02] Let me start with the different types of search that we have been talking about. The first one is the keyword-based search. Most search engines today use keyword-based search. So you fire a query that will have certain keywords and they will try to find it in the index. And whichever document has those keywords, will come out and you will see them.
[00:01:29] But then there is a neural and semantic search. What do we mean by that? Neural and semantic searches don’t rely on keywords, but they mainly focus on the meaning of those keywords, and they try to something that’s similarly found in the index. Let’s take an example to understand.
[00:01:48] Let’s say I want to eat Italian food tonight, and that’s my query, in Google, that’s what I’m typing. How would neural search and semantic search help here? A neural search will [00:02:00] understand I am looking for an Italian restaurant, and based on my current location, it may suggest certain restaurants around me, but semantic search will go one step further in addition to what neural search date.
[00:02:17] It’ll also try to understand what has been my preferences about the restaurant. About the type of Italian food. I also like the intention of the semantic search is to make my wish come true. And depending on that, it’ll try to get me the relevant results on how are implemented behind the scene.
[00:02:38] We, look into that very shortly. Chatbots and conversational, they both try to make it possible for humans to interact with computers in a conversational manner. Chatbots are more kind of static. They produce more kinds of static responses, whereas when you talk about [00:03:00] conversational AI, they understand the human language and the response that they produce is also something that’s very natural and we all looked chatGPT is a wonderful example of a conversational AI agent.
[00:03:15] When we talk about vectors and invents machines and machine learning algorithms, they love to work with numbers, they struggle to work with text, and that’s why the very first thing that we do is that we convert these words into numbers. A set of words, converting it into numbers is something that we call a vector.
[00:03:41] And when you look at words which appear in a similar context, put together, that’s what we call an embedding. What does it mean if we are mentioning a mouse? A relevant word would be cheese or a trap, right? [00:04:00] So you will see a set like, you know, an area of words. Or numbers representing a cat, sorry, mouse cheese and trap all appearing together.
[00:04:12] That’s what we call ambit when we talk about deep neural networks. A neural network is one type of machine learning algorithm which has a structure and function similar to our brain. On a day-to-day basis, we receive so much input through our senses and we process it bit by bit. Our brain processes it bit by bit, and it makes certain decisions based on that.
[00:04:42] When we talk about the neural network, it has a similar structure. It has something we call neurons or nodes. And they also do exactly the same thing. They take inputs from outside, they process it bit by bit to come to some sort of [00:05:00] decision. How this neuro network works we’ll see later on.
[00:05:04] But that is what we need to understand that it is similar to our brain and it is processing this information bit by bit to come to some sort of. Finally, when we look at generative AI and transformers, as the name suggests here, generative AI, it’s a type of artificial intelligence, which helps generate new things.
[00:05:26] It may be a text that it’s generating, it may be an image, it may be a new video, anything… artificial intelligence that generates this new thing. We call it generative AI. Transformer is one type of neural network technology, which help generate this content. They have a special architecture which basically understands the context around the task and it’ll produce more relevant new content.
[00:05:59] What are the [00:06:00] differences between keyword-based neural and semantic search? The techniques which are used, so as we said in keyword based, it’s mainly keyword matching. There are some standard algorithms which are available in engines like, you know, solar elastic search. When you download and when you start using them, that’s what you are getting. Algorithms like tf-idf, and BM25, are some of examples. To make it a bit more powerful the developers would supply some additional information to it, like in additional synonyms, or they will understand how to process the inflexion.
[00:06:36] When we talk about neural search, it’s a type of neural network, and it tries to understand the relations between the different words and phrases, which are appearing in a document. This is what they use. The similarity-matching technique
[00:06:50] is something, that they use at the query time to find out the relevant results.
[00:06:55] So if I say I want for example a jacket for rain, [00:07:00] if in the description it says that, you know it’s good for a wet day, it would understand that because semantically they are similar here. Semantic search is more established. It has been used, it does not rely on the complex technology as the neural network.
[00:07:18] But there are several different ways how you can achieve semantic tech technology. But it’s more about natural language processing, and how you under, how do you parse the data that’s given. For a keyword search, the data requirement is very simple. You can supply just some documents and it’ll start working.
[00:07:37] For neural search, you actually need a lot of data because that’s where you are trying to find out the different types of patterns between the words, so you need a large amount of data. Semantic search can work on a small amount of data as implementation, keywords based search is straightforward.
[00:07:58] Neural search [00:08:00] These days, it has become very easy as well. If you have a lot of data there are quite a few open source libraries you can use to implement a neural search. , yeah. The applications where these different types of searches can be used. So where you have short and simple queries, the keyword search is, is relevant.
[00:08:17] But where you have complex use cases, that’s where you would use actually neural search and semantic search. We understand this with an example. Let’s say a customer fires a query, a dark-coloured dress for a wedding. Now, which search will do what? If it’s a keyword-based search, it’ll remove. The first thing it’ll do is it’ll remove the stop words and it’ll search for dark-coloured dress weddings.
[00:08:44] And I guarantee you, if you’re just using the wedding search, it’ll mess up your result completely. But if you’re using a neural and the semantic search semantic search will help first find out what it is that the customer is looking for. So that will be [00:09:00] dress dark color will be interpreted and the colors like black, blue whale come out in the result.
[00:09:10] But there are still several questions to be answered, just based on that query. You cannot just get the right products. What are these questions? So what’s the size, what’s the gender, and what sort of budget a customer has? This is where a conversational agent can actually help by asking these questions, getting these answers, and then get the results out.
[00:09:39] How do we develop systems like this? We have come a very long way. This image was taken back in 2005. Now this is where we were trying to implement a machine learning algorithm, and we were, we were preparing data for that. The objective was, can we [00:10:00] annotate?
[00:10:04] In the text and we started thinking like, you know, okay, how would somebody write a date? So we started thinking like, you know, okay, these are the ways somebody will write, what are the days, Monday to Sunday? What are the months, January to December? How do people write it? And then we came up with a pattern where we say, day followed by month, followed. We annotated this entire thing and we gave that data to the machine. And we told machine, machine you look at the context and learn how the dates are appearing. This is what we did at that time. Again there was another way of recognizing similarities in the text. We used to use something called LSA, which is Latin Semantic Analysis.
[00:10:51] This was mainly about doing some sort of statistical analysis where we were looking at the documents which had [00:11:00] similar types of words appearing in them. And based on that, we would identify, whether the two words are related. But then in 2013, something, an algorithm called Word to whack I think you might have heard about it.
[00:11:16] That algorithm came and that was based on the neural technology and it started understanding concepts like the cat is two kitten dog is two poppy. So without understanding how they are related, it is understood that you know, these relations have some similarity in them. The similarity invents, they play a very important role in many different applications like search and clustering recommendations.
[00:11:43] So when we talk about recommendations, what we are doing, basically, if you’re looking at a product, we are trying to find other products which are similar to this product, and how do we do that? Well, behind the scene, we are creating these embeddings about that one product and trying to find [00:12:00] other products which have similar meaningful words there.
[00:12:06] When we talk about the building, the deep neural network, this is the simplest image I could find, which explains the neural network. What it basically does?
[00:12:16] So usually, I mean this is a two-dimensional image here, but if you think about our brain the notes here or the neurons here they are connected with each other. Let’s take an example. So we want to predict a weather forecast, right? So some input we are taking like temperature humidity pressure and some other parameters which are related to the prediction of weather forecasting.
[00:12:44] The first set of neurons, they will look at what the temperature is, and they will also look at what’s let’s say the humidity. And based on that, they will predict something that, today’s going to rain or not. This input their own decision. They will actually hand it [00:13:00] over to the next set of neurons and it’ll tell them: Hey, now you process. Now you analyze the other parameters that you have with the result that I’ve given to you, and try to predict is it going to rain or not. Let’s say the second set of neurons predicts dictate that yes, it’s going to rain, but in our training data if it says that, no, it’s not going to rain today, that’s not is a correct answer. This feedback is then given back to all these neurons and they are told, Hey, you have to change something about your prediction.
[00:13:37] Now this neuron this node will change its prediction, they will give it to the next layer again. This process continues until all of them together have predicted the right result. So this is a repetitive process, which is [00:14:00] trying to change their own way of predicting things until they become successful in predicting it correctly.
[00:14:15] This is all very complex, isn’t it?
[00:14:21] Welcome to the modern AI. With modern AI we don’t have to think about any of these things, and this is where we are living today. What modern AI has to offer to us, all of these complex things already made available to us. There are some wonderful libraries available, like Hugging Face TensorFlow, and PyTorch, all of this libraries.
[00:14:48] They have already built so many such models hiding all the complexity behind them, and you can very easily use them. How you can do that? [00:15:00] Let’s have a look at, for example, if you want to do sentiment analysis. What is sentiment analysis? We are basically trying to, let’s say a customer gave a feed feedback and we are trying to understand whether that’s good feedback, bad feedback, or just new normal feedback.
[00:15:19] The number of lines that you have to write these days is just these three lines to do the sentiment analysis. You provide the input and it’ll tell you whether it is negative or positive. Another task is if you want to generate a text. So let’s say, you know, you are writing your emails and you are writing a few words, and the client itself, the Gmail client will actually propose to you what to write next. How is that happening? It’s again, a model like this. You give just a bit of text, and based on those [00:16:00] models, it is trying to propose to you what should be the next text. Again, that’s just four lines of code, as you can see on the screen.
[00:16:15] Question answering with chatGPT. That’s what we are doing, right? We are asking questions and chat GPT is coming back with the answers. How is it predicting those things? Well, it’s one type of training that is done. Let’s look at the chatGPT training here. How did they do this? So they took 7,000 unpublished books and a lot of Wikipedia articles and they gave to a machine.
[00:16:48] Machine is nothing like a baby. And that machine was just reading that content, just reading it for over a year, one year time. It was just reading all this content and it was trying to make some [00:17:00] sense out of it that how these words are connected, what information is present. That’s like learning a language, right?
[00:17:06] Nobody has to teach, but a child learns when staying with their family, with their friends, they would learn about natural things, right? But after that, like we go to school and our teachers give us a task, and when we do it, we may be doing it correctly, or we may be doing it wrong, and the teacher will react with maybe with some negative marking, with some anger or maybe some guidance that you know, this is how you should correct this.
[00:17:31] This is what happens inside neural technology as well. So for six months, open AI guys, they actually interacted with the machine. They gave him feedback that you know what you are doing is correct. What you are doing is wrong. And you know, we don’t know, what you have done is how you can correct it, but you have to figure it out.
[00:17:53] What sort of responses they trained it on? That’s what we call prompt, right? So they, they taught them things [00:18:00] like, you know how to write an. Or how to solve a problem. These were the type of examples. They gave it to this neural machine. And the machine automatically has learned over the 1.5 years of period how to understand all these questions answering things.
[00:18:21] We all have used child, and we all know how powerful that is, but there are certain limitations to as I said, there were certain people who actually gave the feedback to this, and these people might have their own understanding. These people might have their own biases. How they have given the feedback is what we will get answers from this chat GPT3.
[00:18:46] So even though it’s wonderful, it’s very powerful, but we have to be cautious when we use them and we should be making our own decisions whether what it is producing is correct or not. At least at this stage, they [00:19:00] say chat GP3 the GPT4 four version has become a lot more powerful. It’s addressing all these different issues, but still, I think we are in a very, very early stage of utilizing this technology.
[00:19:13] So we have to be very, very powerful. Another limitation here is the machine, which is producing this answer, it doesn’t know what it is doing. That’s why, that’s another reason you have to be very careful in how you use this. And similarly, there are several other limitations to it, and because of that it re still requires a lot of research and, we just look forward to it.
[00:19:41] But what has come out, we know it, it is immense, immensely powerful. And there are certain applications where this can be used. For example, e-comm when we talk about enriching our catalogues, which we have been doing for the last seven years. The introduction of this powerful open AI algorithm [00:20:00] is helping us to make it even better.
[00:20:04] Text two image I don’t know if you have tried a system like DALL-E 2 which given some description, generates some wonderful images.
[00:20:13] So if we look at the industry currently, we know chat GPT. That’s the one system we know, but there are hundreds of such models available. Hugging Face company has more than a hundred thousand models as of yesterday in the repository built by open source community. There is some unicorn company status.
[00:20:37] Companies with unicorn status, Open AI, and Hugging Face, are some of the companies which have done wonderfully well in this area. So here we are and what will happen in the future? This is my take on this. I may be completely wrong but I feel that it’s not [00:21:00] one model world there are a hundred thousand models available in the depository, and each of them is doing something different, something wonderful.
[00:21:11] The future is going to be where we combine all these models together and try to make something really wonderful. Imagine a robot which has not only the power of chatGPT but also understands how to analyze images. How to combine that knowledge with the textual interpretation. And that’s what we, we saw an example in GPT4, the chatGPT that’s the latest release. As we discussed security the information that it’s producing, it’s something to be careful about. Because of that, I personally feel that in the coming days, there will be a lot of investment happening in that area of AI security.[00:22:00]
[00:22:00] And finally, we are in the era, where I was reading some articles and listening to some videos, they were saying that in the next few years, there will be only two types of companies. One would have adopted all these technologies and the second, which does not exist because they haven’t adopted this technology.
[00:22:24] At the least. What we can do is learn to do the prompt engineer. These tools are amazing. We need to know how to ask questions to these machines to get better use of them. And finally, the future begins here. We are using these technologies. Behind the scene as Nilay said, we have been using this, we have been building something like that.
[00:22:50] But the use of open AI has helped us speed up our development. And today we are going to launch something we call which is “hello” in [00:23:00] Finnish. And it’s a shopping conversational AI agent. Thank you.